Making the Crowd Wiser
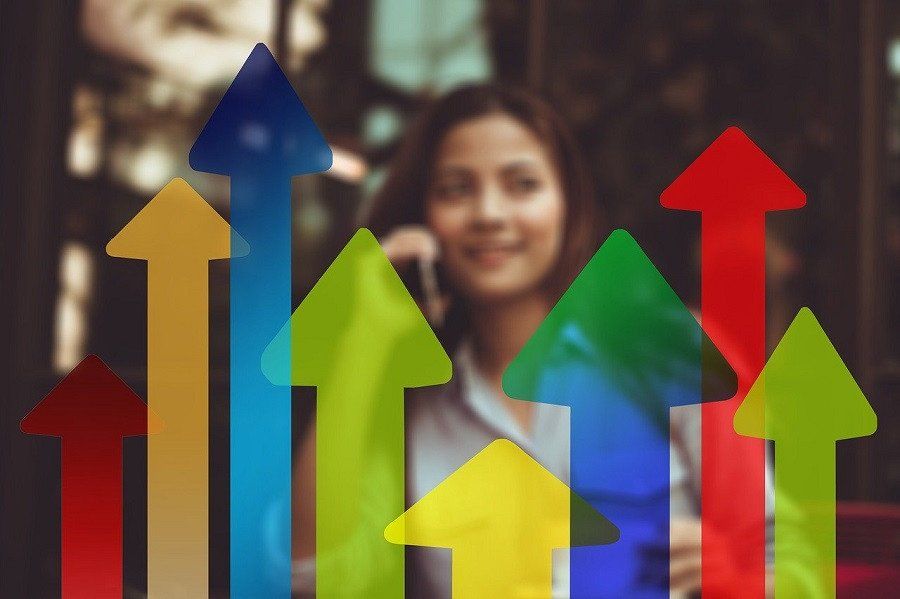
Tools that use averaging to make future predictions might be leaving a lot of relevant information on the table.
How do we measure the chances that a new product like the first Apple Watch will be a success or that a massive project like the Three Gorges Dam will be completed on time? Forecasting these events usually requires either historical data or human experts.
Past information, however, is not always available and is unlikely to be an accurate representation of the future. And, while human experts can adapt to new conditions, they can be expensive and error-prone, not to mention that they often disagree.
The underlying reasons for disagreement among experts are broadly categorised as varying information sources combined with different interpretations and ‘noise’. Experts have different information sources, so they produce a range of forecasts.
Unfortunately, people are not perfectly logical consumers of information:
Experts are susceptible to noise, arising from mood swings, over-optimism or pessimism, or simply misinformation.
A selection of different forecasts is typically summarised with an average. This is problematic because averaging is designed for error reduction.
The assumption is that all experts use the same information and that all disagreement among their forecasts is due to noise. But this isn’t the case.
Harnessed properly, a variety of views brings different insights with the potential for improved forecasting accuracy.
This is not to say that averaging is not useful. It’s simply a tool for a specific task, namely noise reduction, and it should be used for that task only.
There are two accessible approaches that can eliminate the noise of multiple experts without leaving good information on the table: basing a forecast on a common ‘folder’ of information accumulated by experts or extremizing.
Common folders of information
One alternative to averaging alone manipulates experts’ information before averaging their forecasts. We know that averaging works well when the experts have roughly the same information; the key is to organise all experts’ information into a single common folder of information before asking each expert to make their forecasts based only on that information. In theory, the resulting forecasts are all based on the same information so they can be safely averaged.
This concept is illustrated in Oliva and Watson’s case study, “Managing Functional Biases in Organizational Forecasts: A Case Study of Consensus Forecasting in Supply Chain Planning”. It examines a consumer-electronics firm, dubbed ‘Leitax’, where three departments – sales, operations and finance – created three different forecasts.
At first, the information sharing at Leitax was haphazard. Staff members shared spreadsheets between departments, but different variables were embedded in these department-specific documents without any clarification. Other information was shared by word of mouth in the hallways or in the break room.
To make the most of the existing prediction talent at the firm, Oliva and Watson proposed an improved forecasting process. Its design elements addressed the existing incentive misalignments and unintentional departmental biases – like gaps in procedures and informational blind spots that affected some departments.
The improved process establishes an independent forecasting team that:
- asks the sales, operations and finance departments to organise their relevant information according to a predefined set of norms and then merges all these data into a common folder of information
- asks experts from sales, operations and finance to create their own forecasts based on the common folder of information
- combines these forecasts using a weighted average, where the weights are based on how accurate the department’s forecasts have been in the past
To avoid incentive misalignment, the independent forecasting team should operate apart from the other departments and be rewarded based on forecasting accuracy alone. The final weighted average it creates is designed to minimise biases within each department.
Biased forecasting leads to poor performance, which ultimately leads to minimal influence on the company’s aggregate forecast and decision making.
Therefore, a department that wants to continue having influence in the company’s decision making should try to eliminate any biases in its forecasts.
This process based on a common folder of information was highly successful at Leitax. Its forecasting accuracy improved from 58 to 85 per cent; the inventory turns increased from 12 to 26; and inventory was reduced from US$55 million to US$23 million, allowing for quicker reactions to market changes.
Even with these results, the process comes with two caveats. Its success relies on the assumption that all experts are able and willing to make forecasts on all the data in the shared folder.
However, operations may not understand the true relevance of the information added by marketing or HR so their forecast won’t reflect all the information. Furthermore, since each department has its own agenda, sharing information in the common folder comes with the risk of manipulation.
This might interest you: No Reason Why Business Leaders Cannot Tap Into A.I.
A department could purposefully input false information and mislead other departments to increase its own relative weight in the final average. To ensure trust in the common folder information, the independent forecasting team must verify the validity of the data.
Therefore, the involvement of the forecasting team is crucial for ensuring confidence and hence the success of the process. This team is, however, an expensive middleman that can slow down the process or even create bottlenecks in the system.
What if there were a faster and less expensive process to avoid these problems?
Extremizing the average
An alternative approach is a recent technique called extremizing. Unlike the common folder of information approach, extremizing first reduces noise with averaging and then re-introduces the information lost by nudging the average away from the historical base rate.
First, average your experts’ predictions. It may seem counter-intuitive to say averaging isn’t the best tool to keep varied information within a forecast and then suggest it – but averaging is just the first step in this process.
The second step invokes the base rate, the prediction that one would make without any case-specific information. Academic literature refers to this as Bayesian updating; it illustrates how a perfectly rational expert, with a judgment devoid of noise, would process information and forecast the future.
Bayesian inference. Image source: https://www.researchgate.net
Experts without external information forecast the base rate, but as they acquire information specific to the current task, their forecasts begin to deviate from the base rate. In particular, the more informed the experts are, the closer their forecasts are to either 0 or 100 per cent.
They are completely certain an event will or will not come to pass. As an over-the-top example, imagine a fully informed expert to be the equivalent of a person who has a crystal ball, can read the future perfectly, and hence would always forecast either 0 or 100 per cent, depending on whether the event happens or not. This is why we expect to find less informed predictions nearer to the base rate and more informed ones further away from it.
With the logic of Bayesian updating, lost information can be re-introduced by nudging the average away from the base rate and closer to the ideal aggregate.
This process allows experts from different departments to continue making their own independent forecasts without any risk of cross-department manipulation or the additional cost and informational bottleneck of a specific forecasting team. Only the aggregation mechanism must change.
Biases native to silos can be minimised by using a weighted average. The optimal base rate and the degree of extremization are estimated from past forecasts. That is, the historical rates of success/failure indicate the base rate and the degree of extremization is the amount of ‘nudging’ that would have maximised the accuracy of the past average forecasts.
Empirical evidence for extremization comes from a forecasting tournament, hosted by the Intelligence Advanced Research Projects Activity (IARPA) between 2012 and 2016. This pitted forecasting teams against one another to find the most accurate forecasts for hundreds of potential geopolitical upheavals (in finance, politics, economics, etc.) followed by the US intelligence community.
The Good Judgment Project (GJP), led by UPenn’s Barbara Mellers and Philip Tetlock, recruited thousands of experts who made over a million forecasts during the tournament, resulting in one of the largest dataset ever collected in human forecasting.
Extremizing improved the accuracy of the GJP’s final forecasts by an impressive amount. In fact, the development of the extremizing aggregator was one of the main reasons why the GJP outperformed other university teams by 30-70 per cent. Its final predictions were more accurate than prediction markets and even beat intelligence analysts who had inside information.
Prediction choices
To harness the true power of predictions, the goal is to combine many predictions into a ‘super’ consensus that truly reflects all the information from the crowd.
Looking over possible forecasting scenarios, to best harness the knowledge of executives across functions in a business, we shouldn’t average their individual predictions because we don’t want to lose unique information.
Instead, our choices are to either establish a forecasting team that asks all departments to make predictions based on a common folder of information or learn to extremize our forecasting averages. The bottom line is: Do not simply average predictions in business. You can do better!
Collaborator Description:
Business
Tags: Executing Leadership